POLARIZATION AND ECHO CHAMBERS IN #LEYDEEUTANASIA DEBATE ON TWITTER
Abstract
Political dialogue taken to extremes and the positioning of political actors on the margins of the debate is one of the main concerns of citizens in Spain (CIS, 2020). Far from being a phenomenon exclusive to the political class, this distancing is reflected among citizens themselves who, driven by the characteristics of social networks, have developed a special tendency to listen to themselves and avoid connecting with divergent opinions (Sunstein, 2003). The main objective of this study is to analyze the polarization on Twitter around the #LeydeEutanasia debate in Spain. For the analysis of this phenomenon, NodeXL software has been used for data collection and Gephi for its visualization. In addition, an emotional framing table (Menseres, Del Campo & Rueda-Zárate, 2018) has been recreated to analyze the Tweets of Spanish political actors and study their content. In this way, the research has demonstrated the presence of two echo chambers or polarized groups around the debate, which are in line with the ideological blocks on the left/right axis, and which are also observed in the language used to expose their position. However, offensive language is not used in the majority of interactions with the ideological adversary. These data reaffirm the results of previous work in this field of study.
LA POLARIZACIÓN Y ECHO CHAMBERS EN EL DEBATE DE LA #LEYDEEUTANASIA EN TWITTER
Resumen
El diálogo político llevado a los extremos y el posicionamiento de los actores políticos en los márgenes del debate es una de las principales preocupaciones de los ciudadanos en España (CIS, 2020). Lejos de ser un fenómeno exclusivo de la clase política, este distanciamiento tiene su reflejo entre la propia ciudadanía que, impulsada por las características de las redes sociales, ha desarrollado una especial tendencia a escucharse a sí misma, y a evitar conectar con opiniones divergentes (Sunstein, 2003). El objetivo principal del presente estudio es analizar la polarización en Twitter en torno al debate de la #LeydeEutanasia en España. Para el análisis de este fenómeno se ha utilizado el software NodeXL para la recogida de datos y Gephi para su visualización. Además, se ha recreado una tabla de encuadre emocional (Menseres, Del Campo y Rueda-Zárate, 2018) para poder analizar los tuits de los actores políticos españoles y profundizar en su contenido. De esta manera, la investigación ha demostrado la presencia de dos echo chambers o grupos polarizados en torno al debate, que se ajustan a los bloques ideológicos en el eje izquierda/derecha, y que también se observan en el lenguaje utilizado para exponer su postura, en el que sin embargo no se utiliza de forma mayoritaria un lenguaje ofensivo en las interacciones con el adversario ideológico. Estos datos reafirman los resultados de trabajos previos en este ámbito de estudio.
POLARIZAÇAO E ECHO CHAMBERS NO DEBATE DA#LEYDEEUTANASIA NO TWITTER
Resumo
O dialogo politico levado aos extremos e o posicionamento dos atores politicos nas margens do debate é uma das principais preocupações dos cidadãos na espanha (CIS,2020). Longe de ser um fenômeno da classe política, este distanciamento, tem seu reflexo entre a própria cidadania que, impulsionada pelas características das redes sociais, tem desenvolvido uma especial tendência de se escutar a si mesma, e a evitar conectar com opiniões divergentes. (Sunstein, 2003). O objetivo principal do presente estudo é analisar a polarizaçao no twitter em torno ao debate da #LeydeEutanasia na Espanha.Para a análise deste fenômeno se utilizou o software NEdXL para a coleta de dados e Gephi para sua visualização. Além disso foi retirado uma tabela de enquadramento emocional (Menores,Del campo y Rueda Zarate,2018) para poder analisar os tuits dos atores políticos espanhóis e aprofundar no seu conteúdo. Desta forma, a pesquisa tem demonstrado a presença de eco chambers ou grupos polarizados em torno do debate, que se ajustam aos blocos ideológicos no eixo esquerda/direita, e que também se observam na linguagem utilizada para expor sua postura, na que em sua maioria não se usa linguagem ofensiva nas interações com o adversário ideológico. Estes dados reafirmam os resultados prévios neste âmbito e estudo.
Keywords
Twitter, Social media, Cluster analysis, Polarization, Echo chambers, #leydeeutanasia, Ideological blocks
INTRODUCTION
One of the bases of democracy is the freedom of public opinion, and for society to express its opinion freely, it needs free and true information (UNESCO, -). Until a few years ago, the pillar for that freedom was the written press, television, and radio. Nowadays, however, the way we inform ourselves, debate, and form our opinions has profoundly changed with the arrival of online media and especially social networks (Törnberg, 2018: 1).
Social networks, due to their structure, have the potential to bring citizens closer to the political sphere and its influence (Castells, 2011) as they promote more active citizen participation in political affairs and the creation and dissemination of content (Matakos, Terzi, & Tsaparas, 2017; Rodríguez, Aguilar, & Hung, 2010). Social networks also increase the amount of information we have at our disposal and the variety of options to choose from (Sunstein, 2003: 65). Gathering, in the same space, individuals from different countries and cultures who can expand their horizons with new points of view (Papacharissi, 2002: 23).
However, even those tools that offered the opportunity to democratize social relations and bring together people in the discussion who would hardly come into contact without them, have shown areas of weakness when it comes to meeting the created expectations (Bakshy et al., 2015: 1130; Vaccari et al., 2016: 2; Törnberg, 2018: 1; ) (Borgesius et al., 2016).
Social networks can also be used with other intentions, fostering tension, division, and social fracture, using unverified facts or subjective statements that do not seek more than the simple disqualification of the political adversary’s ideas, or manipulation (Rojas, 2007). And it is that, rather than rational and slow deliberation, social networks work by modulating and distributing an atmosphere, a public feeling, which in turn conditions users, often imposing the majority conditions that circulate online (Maldonado, 2016).
Polarization
As users are mostly related to similar opinions, the opposing poles of public opinion move further away: the network is polarized (Lee et al., 2014: 707). Far from a central point of consensus (Baumann et al., 2020). According to Sunstein (2003: 69): <<Immersed in a continuous debate between users who share a similar line of thought, individuals tend to adopt a more extreme position that accentuates the one they originally had>>.
A network is considered polarized if the users can be divided into at least two highly cohesive subgroups that will possibly have two antagonistic points of view (Guerra et al., 2013: 215). A digital space in which users tend to promote their favorite stories, form concentrated groups (Bessi et al., 2016: 2048), and resist information that does not conform to their beliefs (Törnberg, 2018: 2; ) (Quattrociocchi, Scala, & Sunstein, 2016). This is a potential risk for democracy because, in general, people who tend to filter opposing opinions are precisely those who most need to hear other opinions (Sunstein, 2003: 70; Taber and Lodge, 2006: 765).
Vaccari et al. (2016: 1) have shown that Twitter users are more likely to use social media to participate in the networks they support rather than to challenge the user's views. And in turn, group polarization will significantly increase if individuals consider themselves to be part of a group with a shared identity and a certain degree of solidarity. If this is the idea they have of themselves, group polarization is more likely to happen and will tend to become more acute (Sunstein, 2003: 73).
Even so, according to the research by Barberá et al. (2015: 1539), polarization and segregation vary depending on the nature of the events. In turn, they also observed that the level of participation with other users of different ideologies changes according to the ideological opinion of the user and online conversation.
Echo Chambers
In the latest research, the selective nature of the internet-enunciated by Sunstein (2003: 70)- the so-called echo chambers- has gained interest (Vaccari et al., 2016: 2; Sinderman et al., 2020: 1; ; 2) (Buder, Rabl, Feiks, Badermann, & Zurstiege, 2021). Research has revealed that there is a link between echo chambers and polarization (Tonberg, 2018: 2; Sunstein, 2016: 14; ; Garrett, 2009: 280) (Stroud, 2010).
Echo-chambers are a set of nodes closely connected and inclined to share a common opinion about a given narrative (Törnberg, 2018: 2). The Internet, and especially social networks, often function as echo chambers where individuals are exposed to opinions generally similar to their own and rarely disagree between the interlocutors in the discussion (Vaccari et al., 2016: 2; Bakshy et al. al., 2015: 1130). There is, therefore, a natural tendency in action in social networks, both human and digital, that leads individuals not to make choices when it comes to entertainment and news that challenge their pre-existing vision of the world (Sunstein, 2003: 62).
It has also been seen that personality, age, gender, and ideology factors influence when being affected by echo chambers (Sindermann, Elhai, Moshagen, & Montag, 2020). Anonymity can also be added to these elements because previous studies (Davidson et al., 2020: 7) have found particularly high levels of polarization when group members present themselves relatively anonymously and when importance is given to group identity. Based on this, it is reasonable to consider that polarization is more likely to appear and to be extreme when the fact of being a member of the group is something important and individuals have anonymity (Sunstein, 2003: 74).
Furthermore, researchersTsai, Tao, Chuan, and Hong (2020) after research on retweets and mentions, conclude that, although mentions and retweets can be made to users who are not of the same ideology, the majority of interactions (mentions and retweets) are made among members of the internal group (Tsai et al., 2020: 8).
Continuing with the interactions, Buder et al. have shown that the sentiment of the message, that is, the language used by users, can be the precursor to polarization, and ultimately to the fracture of society (Buder et al., 2021: 7).
Polarization and echo chambers in the #LeydeEutanasia
This changing climate on social media has quickly become a threat and is now considered a significant risk to society (Törnberg, 2018: 2; Matakos et al., 2017: 1841). The internet is serving many to spread extremism, precisely because the frequent exposure of people to an extreme position, coupled with the indication that many individuals support such a position, will affect those exposed and predisposed to believe in it. As a consequence, a high degree of fragmentation will be obtained since different people, who at first did not have very specific and perhaps not very different opinions, end up in completely opposite positions as a result of what they read and see (Sunstein, 2003: 74).
OBJECTIVES
The objective of this study is to analyze polarization on Twitter. To carry out the research, the debate on the Euthanasia Law of Spain voted in the Congress of Deputies on December 17th, 2020 has been taken as an object of study. It is not the first time that Congress has voted on a proposal to legalize Euthanasia, in fact, it was voted and rejected up to four times before its approval on this last occasion (Bueno & Martínez, 2019). The last one just three years ago, in 2018, presented by Unidas Podemos, in a session in which a dignified death law proposal by Ciudadanos was also rejected. The law proposal, which was worked on for months, also generated an important controversy both in the traditional media and among the political parties themselves (Hermida, 2020), so that as expected, the discussion around the approval of the law in the lower house also had a great reach on Twitter, as evidenced by the tens of thousands of tweets written on the subject of the Euthanasia Law, some of which are analyzed in these pages.
METHODOLOGY
The material consists of tweets collected on December 17th, 2020 with the hashtag #LeydeEutanasia through the NodeXL platform. It is a software that collects the latest tweets in strictly chronological order (the latest tweets written on the social network) related to the requested hashtag (Kuz et al., 2015: 206). It has been a widely used program in recent years to collect data on social networks for subsequent analysis ( ; Wu, 2013) (Rim, Lee, & Yoo, 2020; Weber, Garimella, & Batayneh, 2013). In total, 22,986 have been collected, the maximum figure that the software allows being collected in its version for the academy, which allows an adequate sampling to be carried out to analyze the rest of the network participating in this debate through it.
These tweets have been visualized in the Gephi.9.2 software, which is used, on the one hand, to perform the calculation of statistics related to the network such as the density of the graph, its diameter, or the entry and exit level of users (Mcsweeney, 2009), and, on the other hand, it allows, through various algorithms, the calculation of clustering coefficients (Cherven, 2015), which allows visualizing the various groups involved in the debate, classified based on the interrelation between them, both before the debate (who follows whom and vice versa) and the resulting one in the debate itself (the interactions they have between them). This software has been used in several research pieces on the same topic (Gaol, Maulana, & Matsuo, 2020; Gualda & Borrero, 2015). Specifically, the OpenOrd algorithm has been used (ibid., 2015: 35) that helps to generate network graphs very quickly, and is more suitable for very large networks due to the loss of precision for the sake of greater speed.
Of these tweets, 5691 tweets have been randomly chosen using the statistical program SPSS (a sample of 25% of the data) for subsequent content analysis. These tweets have been manually analyzed to determine the perspective of users within this debate. For this, the emotional framing classification of Meneses et al. (2018: 46) has been used. Based on this table, we have formulated the following categories for better adaptation to the objective of the study: support (clearly maintains a favorable position), repudiation/dislike (explicitly rejecting the norm), negative offensive (opposed to the approval of the law but it uses offensive terms for the target person of the message), positive offensive (in favor of the approval but when supporting its opinion, it uses offensive language for other users), informative tweet (it is limited to informing about some aspect regarding the law but without evaluating it), non-aligned (those users who do not clearly show an opinion opposed or favorable to the law), and others (for those tweets that do not fit into any other category).
ANALYSIS AND RESULTS
Graph analysis
Three main groups are reflected in the analysis: the first group –in figure 1 in color red/magenta– located on the ideological left, includes the various left-wing sensitivities and political forces, groups, and the media. In total, this group represents 32.35% of the nodes and 35.13% of the edges. We consider a group to be the sum of several clusters which in turn constitute a set of nodes.
In the second group –in figure 1 in color green– located to the right of the graph, are the groups of the ideological right along with other groups and media, and members and associations of the Catholic Church. They are 33.16% of the nodes and 30.93% of the edges.
And lastly, there is a third group in the lower part of the graph –in gray. Two clusters are mixed in this group. In the first of them, Chilean journalists, politicians, and users are located, who, due to the circumstances of the Andean country, where, at the same time that Spain was debating the Euthanasia law in congress, are also included in the hashtag on euthanasia. In the second cluster, one more group is collected that includes various left-and-right-wing organizations and media. As it is not possible to quantify the weight of each cluster within the group, it has been decided not to count this group in the classification of left and right groups. The Chilean cluster Nº. 354 represents a total of 5.76% of the nodes and 4.30% of the edges. While the other group (Nº. 636) includes various left-and-right-wing organizations and media.
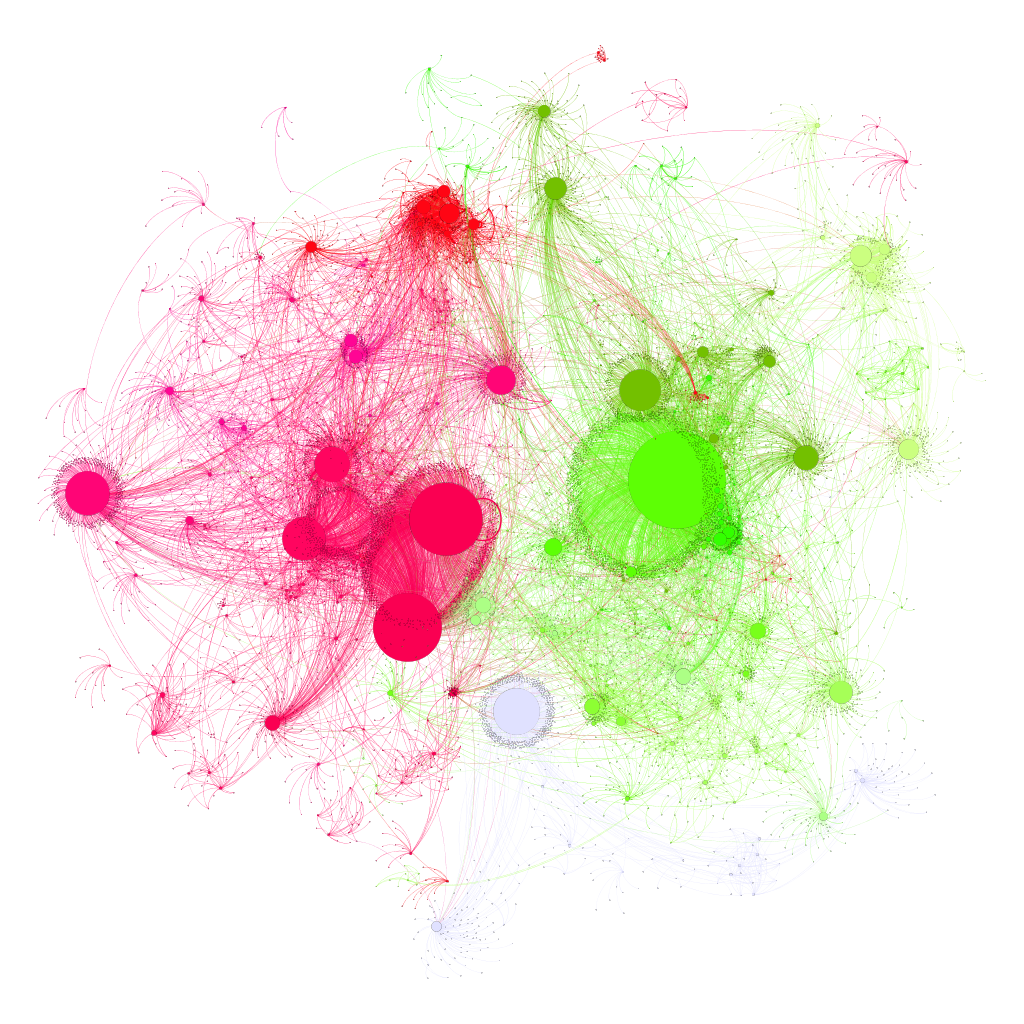
Source: Self-made
The first group known as the ideological left includes 5 of the main clusters. By size in terms of the percentage of nodes in the entire network, from largest to smallest, are the number of the clusters are: 531 (8.98% of total nodes), 2 (7.8%), 1 (7.04%), and 32 (4.79%).
The second group is the one that we classify as the one that includes 6 clusters related to the ideological spectrum of the right: taking into account the percentage of network nodes, from largest to smallest, it includes clusters number: 372 (9.82 % of total nodes), 365 (9.58%), 255 (5.13%), 387 (4.19%), 272 (4.12%), 485 (4%), and cluster 0 (2.55%).
As far as users within these clusters are concerned, it happens that the referential actors of the clusters are mostly users who do not hold public office.
Entry-level
In this section, the users are broken down in terms of the entry-level, which records the number of inquiries received by each of the users that make up the network. The total number of mentions, likes, and retweets received by the user is included among the interpellations.
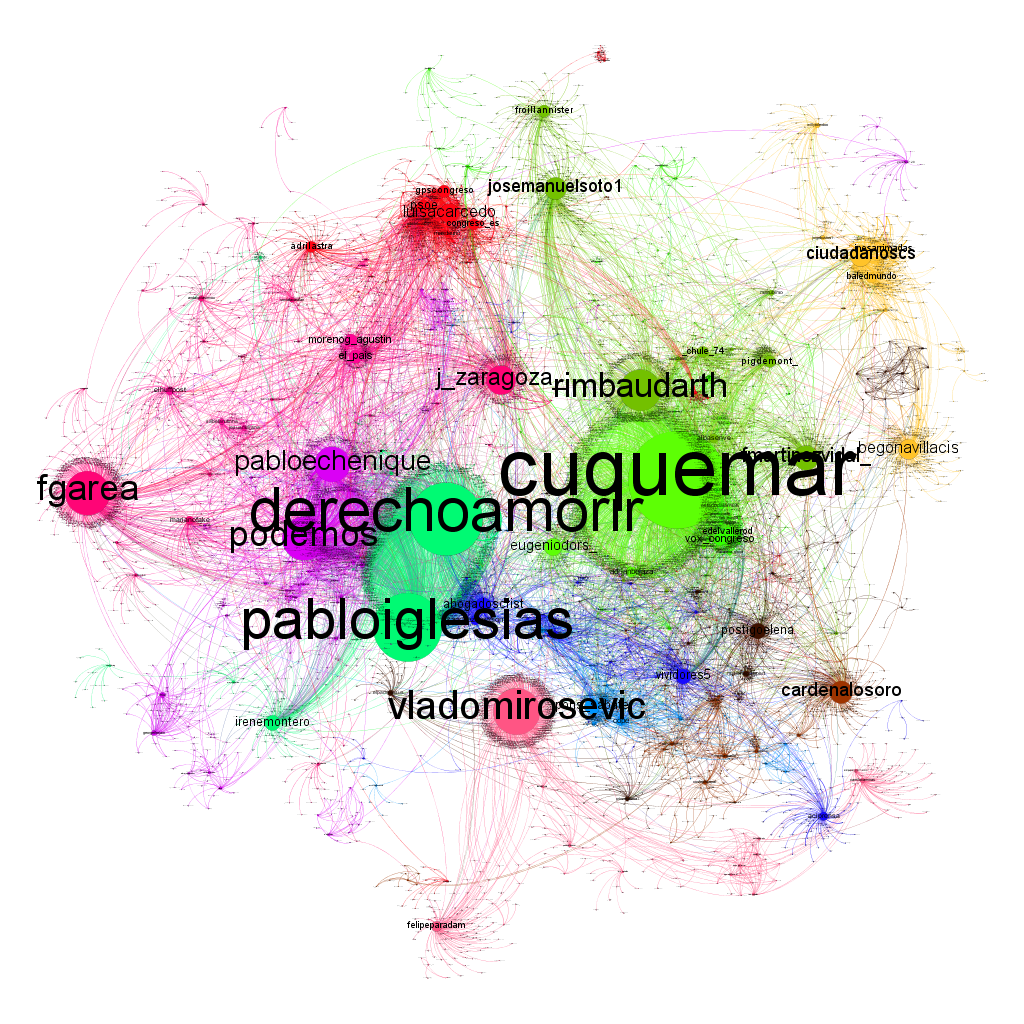
Source: Self-made
In ascending to descending order in terms of entry-level: the largest representative of the network is Marcial Cuquerella (@cuquemar), who defines himself as a media and technology entrepreneur, has an entry-level of 1130, and is a member of cluster nº365. Along with him, in the same cluster, are other Twitter users such as @adrianbelaza -entry-level 113-, or Alberto Asensi (@albasenve) -entry-level 96-.
The next most prominent user is @derechoamorir, the platform Derecho a Morir Dignamente, the association defending the law, with an entry-level coefficient of 842. It is followed by the vice president of social affairs of the Government of Spain, Pablo Iglesias (@PabloIglesias), with a coefficient of 793. This is at the center of the debate, but this does not mean that he has a centralist position, but that Pablo Iglesias is a reference node among the users who make up the position favorable to the approval of the law.
They are followed, in cluster nº531, by Fernando Barea (@Fgarea), deputy director of the digital media outlet El Confidencial (entry-level of 508) and other people who, due to their interactions in the debate, do not appear in the graph directly related to any specific political party in this debate. Behind, cluster No. 2, which corresponds to the Podemos political party (@PODEMOS) -entry-level of 497- and its parliamentary spokesman Pablo Echenique (@PabloEchenique) -entry-level of 402-. This cluster is more related to the political party and its groupings.
In cluster nº372 we find the inverse phenomenon, the main references are people not directly related to a political party @rimbaudarth (entry-level 471) or @josemanuelsoto (entry-level 247), except for the councilor of Madrid of Vox Fer Martínez Vidal (@fmartinesvidal_) with an entry-level of 276.
The next most striking representative is the Archbishop of Madrid, Carlos Osoro (@cardenalosoro) -entry-level of 255-. And next to him are other members of the diocese such as the general secretary of the episcopal conference, Luis Arguello (@MonsArguello) -entry-level of 45-, or the archbishop of Toledo, Francisco Cerro (@Obispofcerro) -entry-level of 39-.
The referential user regarding entry-level in cluster nº272 is the political party Ciudadanos (@CiudadanosCs) -entry-level of 238-, and around it are Edmundo Bal (@BalEdmundo) -entry-level of 117- and Begoña Villacis (@begonavillacis) -entry-level of 224-.
In a new cluster, we find Luisa Carcedo (@luisacarcedo) and several members of the Socialist Party (@PSOE), this group located at the top includes both the political party (with an entry-level of 154) and the parliamentary socialist group and several of its references such as Adriana Lastra (@Adrilastra) -entry-level of 121-, or Odón Elorza (@odonelorza2011) -entry-level of 50-.
Group nº387 is made up of anonymous users such as @eugeniodors, the user with the highest level in the cluster -193-, people from other fields such as Elena Postigo (@PostigoElena), professor of philosophy and bioethics -entry-level 174-, or @princesatesa, with an entry-level of 41.
Cluster nº485 is encompassed by @Vox_congreso, the account of the VOX parliamentary group that has an entry-level of 142, Emilio del Valle (@edelvallerod) with an entry-level of 122, Vox's Deputy in Congress, and next to him there are other politicians and media such as Macarena Ollona (@Macarena_Olona) -entry-level of 48-, Jorge Buxade (@Jorgebuxade) -entry-level of 44-, or the media outlet La Gaceta (@gaceta_es) -entry-level of 48-.
In the last group, nº0, there are several members of the Popular Party, with its president Pablo Casado (@pablocasado_) at the head -entry-level of 18-, although in this group the President of the Government of Spain, Pedro Sánchez (@sanchezcastejon), also stands out. This is because members of the popular party, politically opposed to Sánchez, repeatedly question him in the debate, as can be seen in his high entry-level value -107-.
Table 1. Entry-level Cluster reference users according to their entry-level |
|||
Cluster Number |
Percentage of nodes |
Reference users |
Entry-level |
365 |
9.58% |
@cuquemar |
1130 |
1 |
7.04% |
@derechoamorir |
842 |
531 |
8.98% |
@FBarea |
508 |
2 |
7.8% |
@PODEMOS |
497 |
372 |
9.82% |
@rimbaudarth |
471 |
255 |
5.13% |
@cardenalosoro |
255 |
272 |
4.12% |
@CiudadanosCs |
238 |
32 |
4.79% |
@Luisacarcedo |
219 |
387 |
4.19% |
@eugeniodors |
193 |
485 |
4% |
@Vox_congreso |
142 |
0 |
2.55% |
@sanchezcastejon |
107 |
Source : Self-made
Exit level
We call the exit level the interactions made by the users of the network with the rest of the users, understanding by interactions the mentions, retweets, and likes made to the rest of the users. We must point out that, regarding the exit level, the difference in the number of outstanding users in each group and the rest of the users in the group is much greater than in the entry-level. The graph very visually shows that only a few users from each group participate very actively (they make multiple interpolations towards other users) in the debate. Each of the clusters has one or two users whose activity -visually represented by its size in the graph- is exponentially greater than the rest, clearly standing out visually in the graph. In turn, it should be noted that the classification of the clusters does not vary concerning their size in the general network, so that in this section of the exit level only the users with the highest exit level will be identified, without highlighting the positioning of the cluster.
From largest to smallest concerning the exit level of users, Podemos Alicante Oeste stands out, from cluster nº 2 (@p_alicanteoeste), with 37. Behind it, @ 1denmadrid (cluster nº 1) -exit level 23-, @prensacee from cluster nº 255 with 22, @pilargonzalezl1 from cluster nº 365, @meme_montero from cluster nº 32 and with exit level 17. It is followed closely behind, with 17, by @joselisa17 from cluster nº 372. The next on the list are @martinaguirrecm with 14, @pinkiewarriorpw from cluster nº 272 has the next highest exit level -13-, followed by @juanbasangulo from nº 0 -12-, @jgdeaguilar from nº 387 with 11, and, lastly, @mfedorral from nº 531 with 8.
Table 2. Exit level Cluster reference users according to their exit level |
|||
Cluster Number |
Percentage of nodes |
Reference users |
Exit level |
2 |
7.8% |
@p_alicanteoeste |
37 |
1 |
7.04% |
@1denmadrid |
23 |
255 |
5.13% |
@prensacee |
22 |
365 |
9.58% |
@pilargonzalez1 |
20 |
32 |
4.79% |
@meme_montero |
17 |
372 |
9.82% |
@joselisa17 |
17 |
485 |
4% |
@martinaguirrecm |
14 |
272 |
4.12% |
@pinkiewarrior |
13 |
0 |
2.55% |
@juanbasangulo |
12 |
387 |
4.19% |
@jdeaguilar |
11 |
531 |
8.98% |
@mfedorral |
8 |
Source: Self-made
Emotional framing classification
The analysis of emotional language has revealed that as the image of the Gephi analysis shows, tweets are also divided by language: 2394 tweets (42.06%) show support for the law, and 1993 tweets (35.00%) are against it.
Besides the two main groups, the figure of 242 negative offense tweets stands out, that is, the polarization is high but the language used is not particularly offensive. It is also relevant that 724 analyzed tweets are informative, that is, there is a space for more objective information where aspects related to the law and other calls for activities are explained, such as calls for rallies or similar.
Other less relevant data are the positive offenses 102 tweets (1.79%), the not aligned 8 tweets (0.14%), and, lastly, other 229 tweets (4.02%).
Table 3. Emotional framing |
||
Category |
Amount of Tweets |
Percentages |
Support |
2394 |
42.06% |
Repudiation/Dislike |
1992 |
35.00% |
Negative offensive |
242 |
4.25% |
Positive offensive |
102 |
1.79% |
Informative |
724 |
12.72% |
Not aligned |
8 |
0.14% |
Other |
229 |
4.02% |
Total |
5691 |
100% |
Source : Self-made
DISCUSSION AND CONCLUSIONS
The analysis of the results offers an easily identifiable visual map in which an enormously complex debate is simplified, and in which a large number of factors, actors, and variables interact. Using several techniques, it was possible to identify the most relevant subjects within the object of study, using quantitative techniques, and their subsequent content analysis through a qualitative technique, which allowed the sequencing of tweets and their opinion orientation, in favor or against, regarding the analyzed debate.
Several of the hypotheses formulated at the beginning of the study are confirmed both by the relational graph of the clusters, as well as by the subsequent content analysis and its translation to the emotional framing. On the one hand, the hypothesis that the debates between the different political parties regarding the approval of the euthanasia law, outside of social networks, has a direct bearing on Twitter is validated. And that this discussion does not only take place between the parties themselves or their representatives but extends to other users, as well as to social groups or the media. Furthermore, these results on the ideological/partisan cluster divide on Twitter are also in agreement with the conclusions of Conover et al. (2011:95).
We can also affirm that the network clearly reflects the presence of at least two groups of clusters differentiated from each other in terms of their opinion regarding the debate on the euthanasia law, two prominent echo chambers, which although they have a close relationship between them, and a direct interrelation in the form of edges that unite the nodes with each other, they do not have such close contact with the opposing opinion group, creating a communicative vacuum between them, giving rise to an evident polarization between the opinions favorable and opposed to the approval of the law. The results are consistent with previous studies conducted by: G Garimella and Weber (2017), Vaccari et al. (2016), Q Quattrociocchi et al. (2016), Colleoni, Rozza, and Arvidsson (2014), Hahn, Ryu, and Park (2015), among others.
Furthermore, this is supported by the main idea of Barberá, Jost, Nagler, Tucker, and Bonneau (2015), where they stressed that the nature of the event would change the course of the conversation and polarization and that this would have ideological nuances. Besides the event itself, the phenomenon of polarization also varies from country to country and depending on the circumstances and political conditions of each of them (Urman, 2020). This polarization also occurs in a very even correlation of forces in terms of the percentage of tweets in favor and against, with a narrow margin between users who show their support for the approval of the law, 42% of the tweets, compared to those who oppose it: 35%.
However, it is noteworthy that this structural polarization in the face of the debate does not translate to the same extent to the language used by both groups when interacting with each other. The percentage of favorable and opposing tweets is much greater than those that address the ideological adversary in offensive terms: only 4.25% of the tweets made by users against the approval of the law are written in offensive terms, while those offensive tweets written in favor of the approval of the law are only 1.79%. These results are in line with Buder et al. (2021: 7), pointing out that the language used is a precursor to the division of opinions, marking polarization.
The methodological approach designed for this research offers a static photograph of a discussion that, however, has a dynamic development. Although the study of the debate at the time of the approval of the law offers relevant information about its structure, and the discursive terms in which it occurs, this does not prevent said structure or the discursive framework from being able to evolve or vary over time. Interesting, therefore, are those studies that give continuity over time to the study, to compare the position and forms of the speeches of each other and the motivations behind that change process, to have a greater and more complete understanding about the phenomenon to be studied.
At the same time, the classification of tweets in the emotional frame has been made based on a favorable, contrary, or neutral categorization, without assessing the motivation behind each position. Although throughout the content analysis of the tweets, it has been observed that a large percentage of the tweets in favor denoted the desire for an expansion of social rights, and many of those issued against it argue religious or moral reasons to oppose the approval of the law, it would be interesting to include a methodological analysis that allows classifying the motivations behind each position. The development of these questions raises new research as pertinent on the phenomena of polarization and the motivations that lead to it.